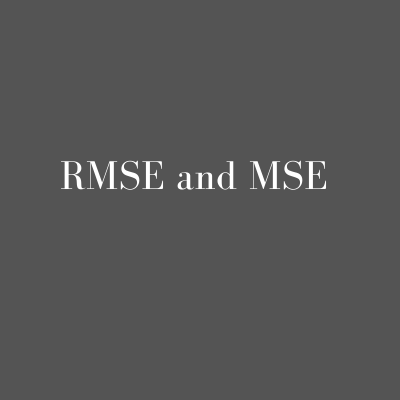
Root mean squared error (RMSE) and (MSE) Mean squared error -
The mean squared error (MSE) and the root mean squared error (RMSE) are two metrics we frequently use to assess how well a model fits a dataset (RMSE).
MSE is a measure that shows the average squared difference between expected and actual values in a dataset. The MSE indicates how well a model fits a dataset. It's a metric for determining how near a fitted line is to actual data points. The closer the fit is to the data set, the lower the Mean Squared Error. The units squared of whatever is plotted on the vertical axis are used in the MSE.
The square root of the average squared difference between the expected and actual values in a dataset is calculated using the RMSE measure. The RMSE indicates how well a model matches a dataset. The RMSE statistic is the easiest to understand because it uses the same units as the quantity represented on the vertical axis or Y-axis. RMSE is a better measure of fit than a correlation coefficient since it can be easily translated into measurement units.
We use the RMSE more often when evaluating how well a model fits a dataset because it is measured in the same units as the response variable. The MSE, on the other hand, is calculated using the response variable's squared units.
Learn what is rmse in details here.
MAE
MAE is less susceptible to outliers than MSE because it does not penalize large errors. When performance is measured using continuous variable data, it is commonly employed. It produces a linear number that equalizes the weighted individual disparities. The model's performance improves as the value decreases.
Conclusion
Here, we learned the difference between root mean squared error and mean squared error and term MAE.
Comments